Регрессионный анализ
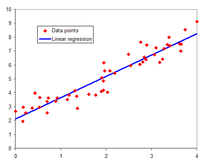
Часть серии о |
Регрессионный анализ |
---|
Модели |
Оценка |
Фон |
Часть серии о |
Машинное обучение и интеллектуальный анализ данных |
---|
В статистическом моделировании регрессионный анализ — это набор статистических процессов для оценки взаимосвязей между зависимой переменной (часто называемой «результатной» или «переменной ответа», или «меткой» на языке машинного обучения) и одной или несколькими независимыми переменными ( часто называемые «предикторами», «ковариатами», «объясняющими переменными» или «признаками»). Наиболее распространенной формой регрессионного анализа является линейная регрессия , при которой находится линия (или более сложная линейная комбинация ), которая наиболее точно соответствует данным в соответствии с определенным математическим критерием. Например, метод обычных наименьших квадратов вычисляет уникальную линию (или гиперплоскость ), которая минимизирует сумму квадратов разностей между истинными данными и этой линией (или гиперплоскостью). По конкретным математическим причинам (см. линейную регрессию ) это позволяет исследователю оценить условное ожидание (или среднее значение совокупности ) зависимой переменной, когда независимые переменные принимают заданный набор значений. Менее распространенные формы регрессии используют несколько иные процедуры для оценки альтернатив. параметры местоположения (например, квантильная регрессия или анализ необходимых условий). [1] ) или оценить условное ожидание по более широкому набору нелинейных моделей (например, непараметрическая регрессия ).
Регрессионный анализ в основном используется для двух концептуально различных целей. Во-первых, регрессионный анализ широко используется для предсказания и прогнозирования , где его использование существенно пересекается с областью машинного обучения . Во-вторых, в некоторых ситуациях регрессионный анализ можно использовать для вывода причинно-следственных связей между независимыми и зависимыми переменными. Важно отметить, что регрессии сами по себе выявляют только отношения между зависимой переменной и набором независимых переменных в фиксированном наборе данных. Чтобы использовать регрессии для прогнозирования или вывода причинно-следственных связей соответственно, исследователь должен тщательно обосновать, почему существующие взаимосвязи обладают предсказательной силой для нового контекста или почему связь между двумя переменными имеет причинно-следственную интерпретацию. Последнее особенно важно, когда исследователи надеются оценить причинно-следственные связи, используя данные наблюдений . [2] [3]
История
[ редактировать ]Самой ранней формой регрессии был метод наименьших квадратов , опубликованный Лежандром в 1805 году. [4] и Гауссом в 1809 году. [5] Лежандр и Гаусс оба применили этот метод к проблеме определения на основе астрономических наблюдений орбит тел вокруг Солнца (в основном комет, но позже и недавно открытых малых планет). Гаусс опубликовал дальнейшее развитие теории наименьших квадратов в 1821 году. [6] включая версию теоремы Гаусса–Маркова .
Термин «регрессия» был придуман Фрэнсисом Гальтоном в 19 веке для описания биологического явления. Феномен заключался в том, что рост потомков высоких предков имеет тенденцию снижаться до нормального среднего значения (феномен, также известный как регрессия к среднему значению ). [7] [8] Для Гальтона регрессия имела только биологический смысл: [9] [10] но позже его работа была расширена Удным Юлом и Карлом Пирсоном на более общий статистический контекст. [11] [12] В работе Юла и Пирсона совместное распределение отклика и объясняющих переменных предполагается гауссовым . Это предположение было ослаблено Р. А. Фишером в его работах 1922 и 1925 гг. [13] [14] [15] Фишер предположил, что условное распределение переменной отклика является гауссовым, но совместное распределение не обязательно должно быть таким. В этом отношении предположение Фишера ближе к формулировке Гаусса 1821 года.
В 1950-х и 1960-х годах экономисты использовали электромеханические настольные калькуляторы для расчета регрессий. До 1970 года получение результата одной регрессии иногда занимало до 24 часов. [16]
Методы регрессии продолжают оставаться областью активных исследований. В последние десятилетия были разработаны новые методы устойчивой регрессии , регрессии, включающей коррелированные ответы, такие как временные ряды и кривые роста , регрессии, в которой предиктором (независимой переменной) или переменными отклика являются кривые, изображения, графики или другие сложные объекты данных. методы регрессии, учитывающие различные типы пропущенных данных, непараметрическая регрессия , байесовские методы регрессии, регрессия, в которой переменные-предикторы измеряются с ошибкой, регрессия с большим количеством переменных-предикторов, чем наблюдений, и причинно-следственный вывод с регрессией.
Регрессионная модель
[ редактировать ]На практике исследователи сначала выбирают модель, которую они хотели бы оценить, а затем используют выбранный ими метод (например, обычный метод наименьших квадратов ) для оценки параметров этой модели. Регрессионные модели включают в себя следующие компоненты:
- Неизвестные параметры , часто обозначаемые как скаляр или вектор. .
- Независимые переменные , которые наблюдаются в данных и часто обозначаются как вектор. (где обозначает строку данных).
- , Зависимая переменная которая наблюдается в данных и часто обозначается скаляром .
- Члены ошибок , которые не наблюдаются непосредственно в данных и часто обозначаются скаляром .
В различных областях применения вместо зависимых и независимых переменных используются разные термины .
Большинство регрессионных моделей предполагают, что является функцией ( функцией регрессии ) и , с представляющий собой аддитивную ошибку , которая может заменять немоделированные детерминанты или случайный статистический шум:
The researchers' goal is to estimate the function that most closely fits the data. To carry out regression analysis, the form of the function must be specified. Sometimes the form of this function is based on knowledge about the relationship between and that does not rely on the data. If no such knowledge is available, a flexible or convenient form for is chosen. For example, a simple univariate regression may propose , suggesting that the researcher believes to be a reasonable approximation for the statistical process generating the data.
Once researchers determine their preferred statistical model, different forms of regression analysis provide tools to estimate the parameters . For example, least squares (including its most common variant, ordinary least squares) finds the value of that minimizes the sum of squared errors . A given regression method will ultimately provide an estimate of , usually denoted to distinguish the estimate from the true (unknown) parameter value that generated the data. Using this estimate, the researcher can then use the fitted value for prediction or to assess the accuracy of the model in explaining the data. Whether the researcher is intrinsically interested in the estimate or the predicted value will depend on context and their goals. As described in ordinary least squares, least squares is widely used because the estimated function approximates the conditional expectation .[5] However, alternative variants (e.g., least absolute deviations or quantile regression) are useful when researchers want to model other functions .
It is important to note that there must be sufficient data to estimate a regression model. For example, suppose that a researcher has access to rows of data with one dependent and two independent variables: . Suppose further that the researcher wants to estimate a bivariate linear model via least squares: . If the researcher only has access to data points, then they could find infinitely many combinations that explain the data equally well: any combination can be chosen that satisfies , all of which lead to and are therefore valid solutions that minimize the sum of squared residuals. To understand why there are infinitely many options, note that the system of equations is to be solved for 3 unknowns, which makes the system underdetermined. Alternatively, one can visualize infinitely many 3-dimensional planes that go through fixed points.
More generally, to estimate a least squares model with distinct parameters, one must have distinct data points. If , then there does not generally exist a set of parameters that will perfectly fit the data. The quantity appears often in regression analysis, and is referred to as the degrees of freedom in the model. Moreover, to estimate a least squares model, the independent variables must be linearly independent: one must not be able to reconstruct any of the independent variables by adding and multiplying the remaining independent variables. As discussed in ordinary least squares, this condition ensures that is an invertible matrix and therefore that a unique solution exists.
Underlying assumptions
[edit]This section needs additional citations for verification. (December 2020) |
By itself, a regression is simply a calculation using the data. In order to interpret the output of regression as a meaningful statistical quantity that measures real-world relationships, researchers often rely on a number of classical assumptions. These assumptions often include:
- The sample is representative of the population at large.
- The independent variables are measured with no error.
- Deviations from the model have an expected value of zero, conditional on covariates:
- The variance of the residuals is constant across observations (homoscedasticity).
- The residuals are uncorrelated with one another. Mathematically, the variance–covariance matrix of the errors is diagonal.
A handful of conditions are sufficient for the least-squares estimator to possess desirable properties: in particular, the Gauss–Markov assumptions imply that the parameter estimates will be unbiased, consistent, and efficient in the class of linear unbiased estimators. Practitioners have developed a variety of methods to maintain some or all of these desirable properties in real-world settings, because these classical assumptions are unlikely to hold exactly. For example, modeling errors-in-variables can lead to reasonable estimates independent variables are measured with errors. Heteroscedasticity-consistent standard errors allow the variance of to change across values of . Correlated errors that exist within subsets of the data or follow specific patterns can be handled using clustered standard errors, geographic weighted regression, or Newey–West standard errors, among other techniques. When rows of data correspond to locations in space, the choice of how to model within geographic units can have important consequences.[17][18] The subfield of econometrics is largely focused on developing techniques that allow researchers to make reasonable real-world conclusions in real-world settings, where classical assumptions do not hold exactly.
Linear regression
[edit]In linear regression, the model specification is that the dependent variable, is a linear combination of the parameters (but need not be linear in the independent variables). For example, in simple linear regression for modeling data points there is one independent variable: , and two parameters, and :
- straight line:
In multiple linear regression, there are several independent variables or functions of independent variables.
Adding a term in to the preceding regression gives:
- parabola:
This is still linear regression; although the expression on the right hand side is quadratic in the independent variable , it is linear in the parameters , and
In both cases, is an error term and the subscript indexes a particular observation.
Returning our attention to the straight line case: Given a random sample from the population, we estimate the population parameters and obtain the sample linear regression model:
The residual, , is the difference between the value of the dependent variable predicted by the model, , and the true value of the dependent variable, . One method of estimation is ordinary least squares. This method obtains parameter estimates that minimize the sum of squared residuals, SSR:
Minimization of this function results in a set of normal equations, a set of simultaneous linear equations in the parameters, which are solved to yield the parameter estimators, .

In the case of simple regression, the formulas for the least squares estimates are
where is the mean (average) of the values and is the mean of the values.
Under the assumption that the population error term has a constant variance, the estimate of that variance is given by:
This is called the mean square error (MSE) of the regression. The denominator is the sample size reduced by the number of model parameters estimated from the same data, for regressors or if an intercept is used.[19] In this case, so the denominator is .
The standard errors of the parameter estimates are given by
Under the further assumption that the population error term is normally distributed, the researcher can use these estimated standard errors to create confidence intervals and conduct hypothesis tests about the population parameters.
General linear model
[edit]In the more general multiple regression model, there are independent variables:
where is the -th observation on the -th independent variable.If the first independent variable takes the value 1 for all , , then is called the regression intercept.
The least squares parameter estimates are obtained from normal equations. The residual can be written as
The normal equations are
In matrix notation, the normal equations are written as
where the element of is , the element of the column vector is , and the element of is . Thus is , is , and is . The solution is
Diagnostics
[edit]Once a regression model has been constructed, it may be important to confirm the goodness of fit of the model and the statistical significance of the estimated parameters. Commonly used checks of goodness of fit include the R-squared, analyses of the pattern of residuals and hypothesis testing. Statistical significance can be checked by an F-test of the overall fit, followed by t-tests of individual parameters.
Interpretations of these diagnostic tests rest heavily on the model's assumptions. Although examination of the residuals can be used to invalidate a model, the results of a t-test or F-test are sometimes more difficult to interpret if the model's assumptions are violated. For example, if the error term does not have a normal distribution, in small samples the estimated parameters will not follow normal distributions and complicate inference. With relatively large samples, however, a central limit theorem can be invoked such that hypothesis testing may proceed using asymptotic approximations.
Limited dependent variables
[edit]Limited dependent variables, which are response variables that are categorical variables or are variables constrained to fall only in a certain range, often arise in econometrics.
The response variable may be non-continuous ("limited" to lie on some subset of the real line). For binary (zero or one) variables, if analysis proceeds with least-squares linear regression, the model is called the linear probability model. Nonlinear models for binary dependent variables include the probit and logit model. The multivariate probit model is a standard method of estimating a joint relationship between several binary dependent variables and some independent variables. For categorical variables with more than two values there is the multinomial logit. For ordinal variables with more than two values, there are the ordered logit and ordered probit models. Censored regression models may be used when the dependent variable is only sometimes observed, and Heckman correction type models may be used when the sample is not randomly selected from the population of interest. An alternative to such procedures is linear regression based on polychoric correlation (or polyserial correlations) between the categorical variables. Such procedures differ in the assumptions made about the distribution of the variables in the population. If the variable is positive with low values and represents the repetition of the occurrence of an event, then count models like the Poisson regression or the negative binomial model may be used.
Nonlinear regression
[edit]When the model function is not linear in the parameters, the sum of squares must be minimized by an iterative procedure. This introduces many complications which are summarized in Differences between linear and non-linear least squares.
Prediction (interpolation and extrapolation)
[edit]
Regression models predict a value of the Y variable given known values of the X variables. Prediction within the range of values in the dataset used for model-fitting is known informally as interpolation. Prediction outside this range of the data is known as extrapolation. Performing extrapolation relies strongly on the regression assumptions. The further the extrapolation goes outside the data, the more room there is for the model to fail due to differences between the assumptions and the sample data or the true values.
A prediction interval that represents the uncertainty may accompany the point prediction. Such intervals tend to expand rapidly as the values of the independent variable(s) moved outside the range covered by the observed data.
For such reasons and others, some tend to say that it might be unwise to undertake extrapolation.[21]
However, this does not cover the full set of modeling errors that may be made: in particular, the assumption of a particular form for the relation between Y and X. A properly conducted regression analysis will include an assessment of how well the assumed form is matched by the observed data, but it can only do so within the range of values of the independent variables actually available. This means that any extrapolation is particularly reliant on the assumptions being made about the structural form of the regression relationship. If this knowledge includes the fact that the dependent variable cannot go outside a certain range of values, this can be made use of in selecting the model – even if the observed dataset has no values particularly near such bounds. The implications of this step of choosing an appropriate functional form for the regression can be great when extrapolation is considered. At a minimum, it can ensure that any extrapolation arising from a fitted model is "realistic" (or in accord with what is known).
Power and sample size calculations
[edit]There are no generally agreed methods for relating the number of observations versus the number of independent variables in the model. One method conjectured by Good and Hardin is , where is the sample size, is the number of independent variables and is the number of observations needed to reach the desired precision if the model had only one independent variable.[22] For example, a researcher is building a linear regression model using a dataset that contains 1000 patients (). If the researcher decides that five observations are needed to precisely define a straight line (), then the maximum number of independent variables the model can support is 4, because
- .
Other methods
[edit]Although the parameters of a regression model are usually estimated using the method of least squares, other methods which have been used include:
- Bayesian methods, e.g. Bayesian linear regression
- Percentage regression, for situations where reducing percentage errors is deemed more appropriate.[23]
- Least absolute deviations, which is more robust in the presence of outliers, leading to quantile regression
- Nonparametric regression, requires a large number of observations and is computationally intensive
- Scenario optimization, leading to interval predictor models
- Distance metric learning, which is learned by the search of a meaningful distance metric in a given input space.[24]
Software
[edit]All major statistical software packages perform least squares regression analysis and inference. Simple linear regression and multiple regression using least squares can be done in some spreadsheet applications and on some calculators. While many statistical software packages can perform various types of nonparametric and robust regression, these methods are less standardized. Different software packages implement different methods, and a method with a given name may be implemented differently in different packages. Specialized regression software has been developed for use in fields such as survey analysis and neuroimaging.
See also
[edit]- Anscombe's quartet
- Curve fitting
- Estimation theory
- Forecasting
- Fraction of variance unexplained
- Function approximation
- Generalized linear model
- Kriging (a linear least squares estimation algorithm)
- Local regression
- Modifiable areal unit problem
- Multivariate adaptive regression spline
- Multivariate normal distribution
- Pearson correlation coefficient
- Quasi-variance
- Prediction interval
- Regression validation
- Robust regression
- Segmented regression
- Signal processing
- Stepwise regression
- Taxicab geometry
- Linear trend estimation
References
[edit]- ^ Necessary Condition Analysis
- ^ David A. Freedman (27 April 2009). Statistical Models: Theory and Practice. Cambridge University Press. ISBN 978-1-139-47731-4.
- ^ Р. Деннис Кук; Сэнфорд Вейсберг Критика и анализ влияния в регрессии , Социологическая методология , Vol. 13. (1982), стр. 313–361.
- ^ AM Лежандр . Новые методы определения орбит комет , Фирмен Дидо, Париж, 1805 г. «О методе наименьших квадратов» появляется в качестве приложения.
- ^ Перейти обратно: а б Глава 1: Ангрист, Дж. Д. и Пишке, Дж. С. (2008). В основном безобидная эконометрика: спутник эмпирика . Издательство Принстонского университета.
- ^ CF Гаусс. Теория сочетания наблюдений допускает мельчайшие ошибки . (1821/1823)
- ^ Могул, Роберт Г. (2004). Прикладная статистика за второй семестр . Кендалл/Хант Издательская компания. п. 59. ИСБН 978-0-7575-1181-3 .
- ^ Гальтон, Фрэнсис (1989). «Родство и корреляция (переиздано в 1989 г.)» . Статистическая наука . 4 (2): 80–86. дои : 10.1214/ss/1177012581 . JSTOR 2245330 .
- ^ Фрэнсис Гальтон . «Типичные законы наследственности», Nature 15 (1877), 492–495, 512–514, 532–533. (Гальтон использует термин «реверсия» в этой статье, где обсуждается размер горошин.)
- ^ Фрэнсис Гальтон. Послание Президента, Раздел H, Антропология. (1885) (Гальтон использует термин «регрессия» в этой статье, где обсуждается рост человека.)
- ^ Юле, Г. Удный (1897). «К теории корреляции» . Журнал Королевского статистического общества . 60 (4): 812–54. дои : 10.2307/2979746 . JSTOR 2979746 .
- ^ Пирсон, Карл ; Юл, ГУ; Бланшар, Норман; Ли, Алиса (1903). «Закон наследственности» . Биометрика . 2 (2): 211–236. дои : 10.1093/biomet/2.2.211 . JSTOR 2331683 .
- ^ Фишер, Р.А. (1922). «Наличие соответствия формул регрессии и распределение коэффициентов регрессии» . Журнал Королевского статистического общества . 85 (4): 597–612. дои : 10.2307/2341124 . JSTOR 2341124 . ПМЦ 1084801 .
- ^ Рональд А. Фишер (1954). Статистические методы для научных работников (Двенадцатое изд.). Эдинбург : Оливер и Бойд. ISBN 978-0-05-002170-5 .
- ^ Олдрич, Джон (2005). «Фишер и регрессия» (PDF) . Статистическая наука . 20 (4): 401–417. дои : 10.1214/088342305000000331 . JSTOR 20061201 .
- ^ Родни Рамчаран. Регрессии: почему экономисты одержимы ими? Март 2006 г. По состоянию на 3 декабря 2011 г.
- ^ Фотерингем, А. Стюарт; Брансдон, Крис; Чарльтон, Мартин (2002). Географически взвешенная регрессия: анализ пространственно изменяющихся отношений (переиздание). Чичестер, Англия: Джон Уайли. ISBN 978-0-471-49616-8 .
- ^ Фотерингем, AS; Вонг, DWS (1 января 1991 г.). «Проблема модифицируемой единицы площади в многомерном статистическом анализе». Окружающая среда и планирование А . 23 (7): 1025–1044. дои : 10.1068/a231025 . S2CID 153979055 .
- ^ Стил, РГД, и Торри, Дж. Х., Принципы и процедуры статистики с особым упором на биологические науки. , МакГроу Хилл , 1960, стр. 288.
- ^ Руо, Матье (2013). Вероятность, статистика и оценка (PDF) . п. 60.
- ^ Чан, CL, (2003) Статистические методы анализа , World Scientific. ISBN 981-238-310-7 - стр. 274, раздел 9.7.4 «Интерполяция и экстраполяция»
- ^ Хорошо, Пи ; Хардин, JW (2009). Распространенные ошибки в статистике (и как их избежать) (3-е изд.). Хобокен, Нью-Джерси: Уайли. п. 211. ИСБН 978-0-470-45798-6 .
- ^ Тофаллис, К. (2009). «Процентная регрессия по методу наименьших квадратов» . Журнал современных прикладных статистических методов . 7 : 526–534. дои : 10.2139/ssrn.1406472 . HDL : 2299/965 . ССНР 1406472 .
- ^ ЯнЦзин Лун (2009). «Оценка возраста человека с помощью метрического обучения для задач регрессии» (PDF) . Учеб. Международная конференция по компьютерному анализу изображений и узоров : 74–82. Архивировано из оригинала (PDF) 8 января 2010 г.
Дальнейшее чтение
[ редактировать ]- Уильям Х. Краскал и Джудит М. Танур , изд. (1978), «Линейные гипотезы», Международная энциклопедия статистики . Свободная пресса, т. 1,
- Эван Дж. Уильямс, «I. Регрессия», стр. 523–41.
- Джулиан К. Стэнли , «II. Дисперсионный анализ», стр. 541–554.
- Линдли, Д.В. (1987). «Регрессионный и корреляционный анализ», Нью-Пэлгрейв: Экономический словарь , т. 4, стр. 120–23.
- Биркс, Дэвид и Додж, Ю. , Альтернативные методы регрессии . ISBN 0-471-56881-3
- Чатфилд, К. (1993) « Расчет интервальных прогнозов », Журнал деловой и экономической статистики, 11 . стр. 121–135.
- Дрейпер, Северная Каролина; Смит, Х. (1998). Прикладной регрессионный анализ (3-е изд.). Джон Уайли. ISBN 978-0-471-17082-2 .
- Фокс, Дж. (1997). Прикладной регрессионный анализ, линейные модели и родственные методы. Мудрец
- Хардл, В., Прикладная непараметрическая регрессия (1990), ISBN 0-521-42950-1
- Мид, Найджел; Ислам, Тохидул (1995). «Интервалы прогнозирования для прогнозов кривой роста». Журнал прогнозирования . 14 (5): 413–430. дои : 10.1002/for.3980140502 .
- А. Сен, М. Сривастава, Регрессионный анализ — теория, методы и приложения , Springer-Verlag, Берлин, 2011 (4-е издание).
- Т. Струц: Подбор данных и неопределенность (Практическое введение в метод взвешенных наименьших квадратов и не только) . Вьюег+Тойбнер, ISBN 978-3-8348-1022-9 .
- Стульп, Фрик и Оливье Сиго. Множество алгоритмов регрессии, одна унифицированная модель: обзор. Нейронные сети, вып. 69, сентябрь 2015 г., стр. 60–79. https://doi.org/10.1016/j.neunet.2015.05.005 .
- Малакути, Б. (2013). Операции и производственные системы с множеством целей . Джон Уайли и сыновья.
- Чикко, Давиде; Уорренс, Маттейс Дж.; Юрман, Джузеппе (2021). «Коэффициент детерминации R-квадрат более информативен, чем SMAPE, MAE, MAPE, MSE и RMSE при оценке регрессионного анализа» . PeerJ Информатика . 7 (e623): e623. дои : 10.7717/peerj-cs.623 . ПМЦ 8279135 . ПМИД 34307865 .
Внешние ссылки
[ редактировать ]
- «Регрессионный анализ» , Математическая энциклопедия , EMS Press , 2001 [1994]
- Самое раннее использование: регрессия - основная история и ссылки.
- Для чего используется множественная регрессия? – Множественная регрессия
- Регрессия слабокоррелированных данных – как могут появиться ошибки линейной регрессии, когда диапазон Y намного меньше диапазона X